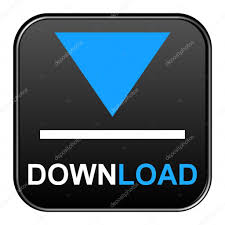
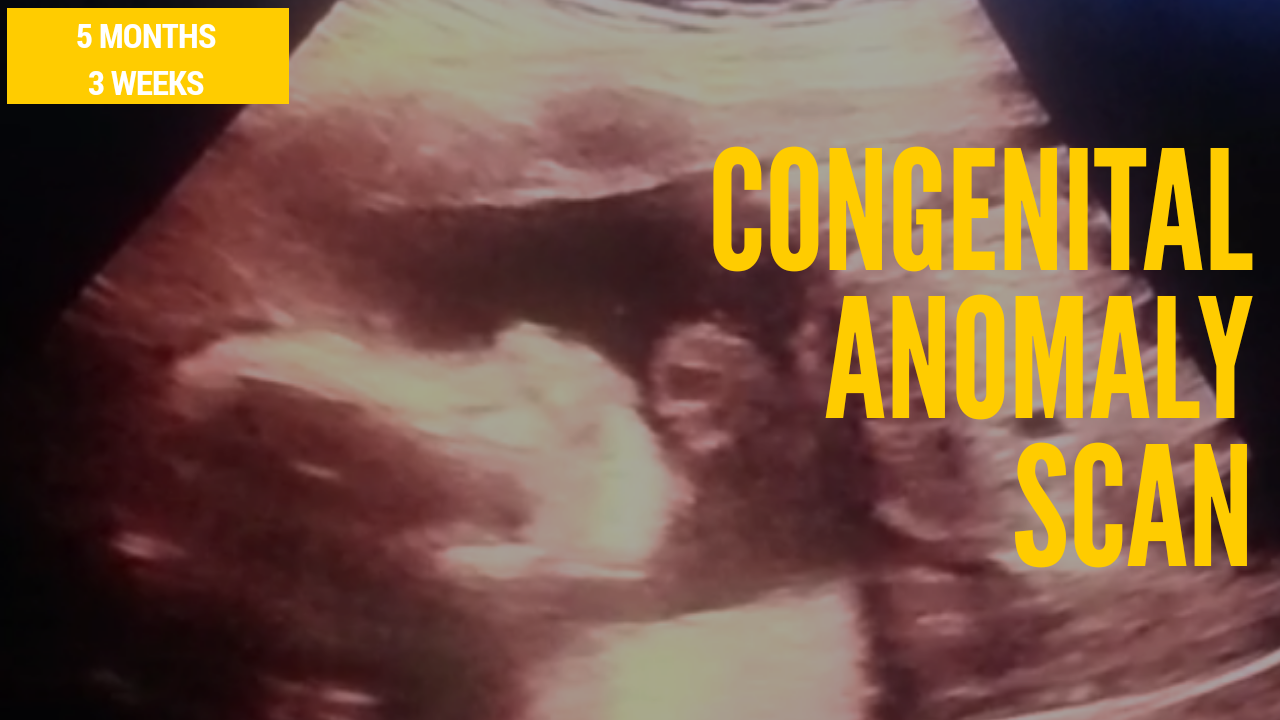
Each event log is separated into traces, and each trace reflects one process instance’s execution. The event log is the main data structure of the business process data that contain information regarding what activities have been performed in a process, who executed them, at which time, etc. Furthermore, business processes are carried out in a variety of flexible infrastructures and networked settings where they play important roles and allow key business activities. Business processes integrate multiple data sources, applications, and information technology systems, and manage public as well as confidential private information. Longer processes tend to have multiple dependencies between the activities. The process can be simple or complex, depending on the number of activities. There is a requirement for an automated system that can discover abnormalities in massive business process logs.Ī business process is a set of activities or tasks triggered by an event to achieve a specific organization’s goal.
#Anomaly meaning manual#
Manual identification of abnormalities in business process data is time-consuming, labor-intensive, and prone to human error owing to the vast volumes of data. On average, organizations have lost a gross profit of 7% annually to fraud. In 2012, the Association of Certified Fraud Examiners reported that there had been 1388 fraud cases in 96 countries, which caused $1.4 billion in losses. The findings revealed that the proposed approach outperformed five competing approaches by efficiently using the few available anomalous examples.Īnomalies in business processes can result in significant losses. Multiple scenarios were used to test the proposed approach on real-world and synthetic datasets. Furthermore, to efficiently model the sequential nature of business process data and to handle the long-term dependences, we used a long short-term memory network combined with a self-attention mechanism to develop the agent of our reinforcement learning model. This approach depends on the proximity between the data samples in the latent space of the variational autoencoder. To further reduce data deficiency, we introduced a sampling method to allow the effective exploration of the unlabeled data and to address the imbalanced data problem, which is a common problem in the anomaly detection field. We created a unique reward function that combined the supervisory signal supplied by a variational autoencoder trained on unlabeled data with the supervisory signal provided by the environment’s reward. The proposed approach is intended to use a small collection of labeled anomalous data while exploring a huge set of unlabeled data to find new classes of anomalies that are outside the scope of the labeled anomalous data.
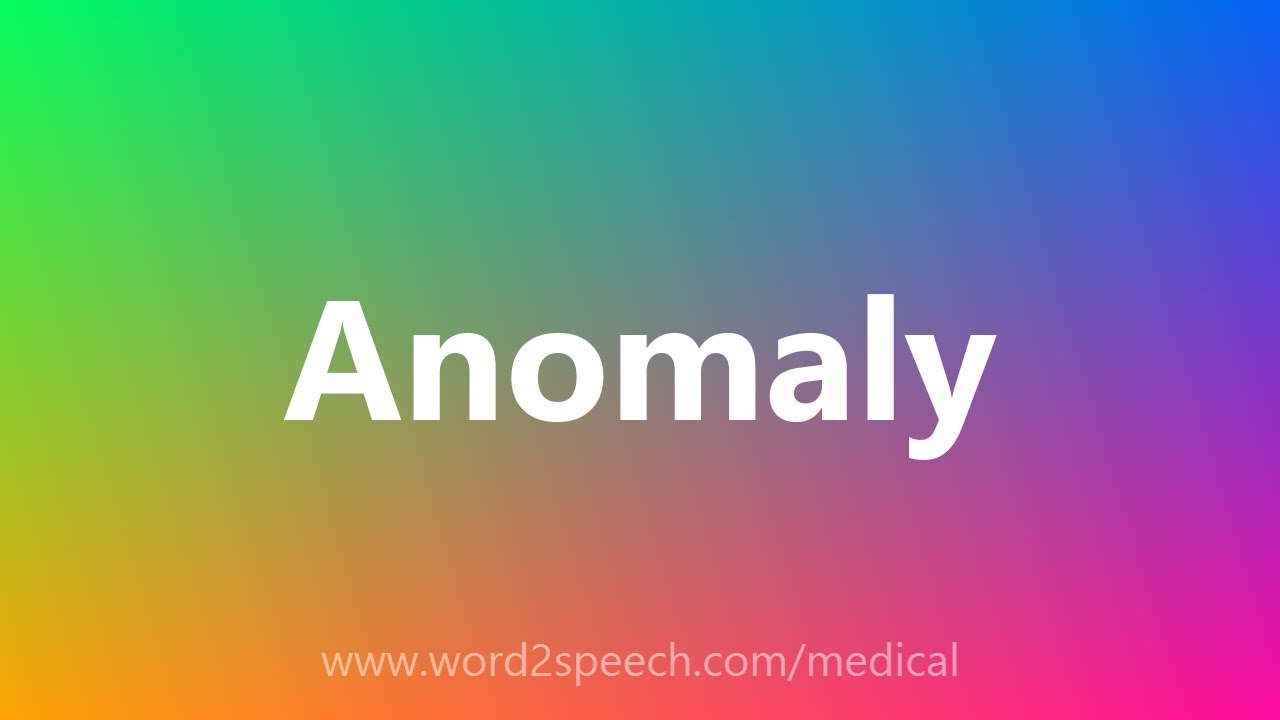
In this study, we propose a deep weakly supervised reinforcement learning-based approach to identify anomalies in business processes by leveraging limited labeled anomaly data. However, these methods do not work well because of the absence of prior knowledge of true anomalies.

For this reason, unsupervised learning techniques and semi-supervised learning approaches trained on entirely labeled normal data have dominated this domain for a long time. Supervised learning techniques are impracticable because of the difficulties of gathering huge amounts of labeled business process anomaly data. The detection of anomalous behavior in business process data is a crucial task for preventing failures that may jeopardize the performance of any organization.
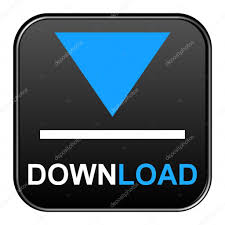